Systematising processes and harnessing technology to automate simple decision-making
The concept of “Noise,” a term coined by Daniel Kahneman, Olivier Sibony, and Cass R. Sunstein in their book Noise: A Flaw in Human Judgment, is a problematic aspect of our decision-making process that I believe should be highlighted and taken seriously. I see the term bounded about quite a bit, and its definition in common parlance seems to have expanded to the extent that it has lost its significance.
Noise is prevalent everywhere there is judgement in industries like medicine, recruitment, the judicial system, and, as we’ll explore in this article, insurance. Unlike bias, which is predictable and systematic, Noise, in essence, is the inconsistency in decisions made by different individuals under similar circumstances or within ‘systems.’
This randomness poses a significant challenge to organisations because it affects employees’ judgments and subsequent business outcomes.
So, how can we tackle Noise and achieve a more consistent basis for decision-making?
The answer lies in systematising processes and harnessing technology to automate simple decision-making. This two-part article will delve into the concept of Noise in the insurance industry, its impact on organisations, and practical ways to leverage technology to reduce Noise and enhance overall organisational accuracy.
What is the Difference Between Noise and Bias?
Before we proceed, let’s differentiate between Noise and Bias. Noise refers to the random, unpredictable variability in judgements that bias cannot account for. Kahneman provides valuable insights on Noise and uses a target analogy to illustrate the difference.
Let’s take five people to an archery range. Everyone uses the same bow to shoot at the same bullseye. Everyone misses the bullseye but hits the same spot at the top-right of the target. What is wrong with the bow? Why is everyone hitting the same place on the target but not the bullseye? Is the wind blowing in that direction? Is the bow strung incorrectly? It must be something. This is bias.
Unlike the randomness of Noise, there is a causal explanation with bias, a reason, or reasons as to why people are making the same error. Most importantly, biases support a prediction. If we repeat the same experiment, we could assume the results will be similar the next time.
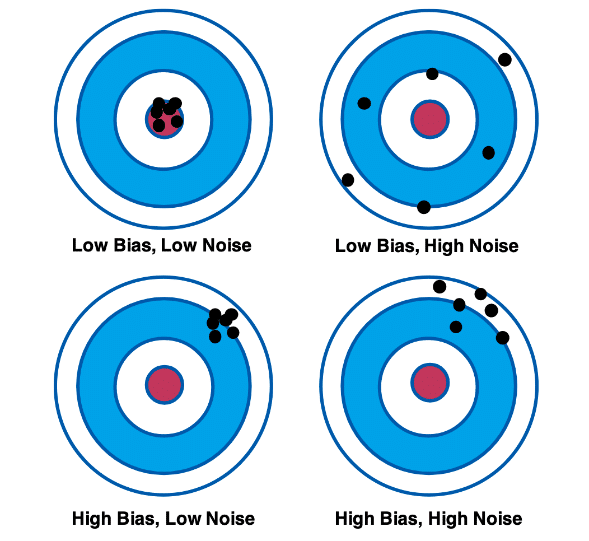
Image Adapted from Source: Kahneman, D., Sibony, O., & Sunstein, C. R. (2021). Noise: A Flaw in Human Judgment. Little, Brown Spark.
To further illustrate this concept, imagine a scenario where two medical practitioners examine the same patient independently. Despite having access to the same information and clinical history, they come to different diagnoses. This discrepancy results from Noise, a randomness or variability in human judgment that leads to inconsistent outcomes. In this situation, variability in a diagnosis is not a desirable outcome, especially for the patient, so we must take measures to reduce its effects.
Understanding Noise in the Insurance Industry
What does Noise look like in the insurance industry? In the insurance context, noise influences the variability of judgments made by underwriters and claims adjusters. These professionals play a critical role in their firm’s success. They must make critical decisions with a high degree of precision and consistency because their judgements bind the company to substantial financial consequences.
Underwriters are responsible for gathering and analysing risk data, measuring risk, and generating premiums. The optimal price, often referred to as the “Goldilocks number,” should be neither too high nor too low. If the premium is too low, the underwriter risks onboarding poor-quality and unprofitable business—a direct contradiction to the purpose of their role. If the quote is too high, the underwriter risks losing business to a competitor.
In an ideal situation, therefore, we expect minimal variance from this number as a matter of utmost commercial importance.
Claims Adjusters gather information to estimate a claim’s value to provide a starting point for negotiation. If the estimate is too high, it may embolden the client to make challenging and potentially nuclear demands. Conversely, a low estimate might result in legal action, reputational damage, and loss of trust. In a highly trust-based industry, (re)insurance firms can ill afford these consequences.
Here’s The Interesting Bit!
A “Noise Audit” conducted by Kahneman and the team revealed that differences in underwriting pricing and claims estimates were far more significant than insurance Executives expected. Perhaps the most striking aspect of Noise is not its existence but the fact that senior leaders are not fully aware of its impact.
When the following question was posed to Executives, “In a well-run insurance company, if you randomly selected two qualified underwriters or claim adjusters, how different would you expect their estimates for the same case to be? Specifically, what would be the difference between the two estimates as a percentage of their average?
Most Executives of the insurance firm guessed 10% or less. Interestingly, when Kahneman posed the same question to over 800 CEOs in other industries, the median and most frequent response was also 10%, while the second most popular guess was 15%.
The audit results, however, showed considerable variability. The median difference in underwriting was actually 55%, indicating a significant impact of Noise in decision-making processes. The audit also revealed similar results among pairs of claims adjusters, with a median difference of 44%. That’s quite significant, what would be the impact if organisations reduced these medians even by 5 – 10%? Simply put, we must take these findings seriously.
Noise is unwanted; it has no benefits and can have substantial financial impacts on an organisation. But the good news is we can do something about it. With awareness comes action. Now that we are aware of the effects that Noise can have within insurance firms, we must further explore the available remedies to Noise, of which there are several!
About the Author:
Tim Hatzis is an accomplished insurance technology expert with 8+ years of solving automation challenges. He currently serves as the Global Reinsurance Lead at Bits In Glass and is considered a thought leader in the field. He is fascinated with psychology, business processes and how technology can be leveraged to support organisations in achieving business success.